Ideas Worth Exploring: 2025-03-18
- Charles Ray
- Mar 18
- 4 min read
Ideas: Alperen Keles - Verifiability is the Limit
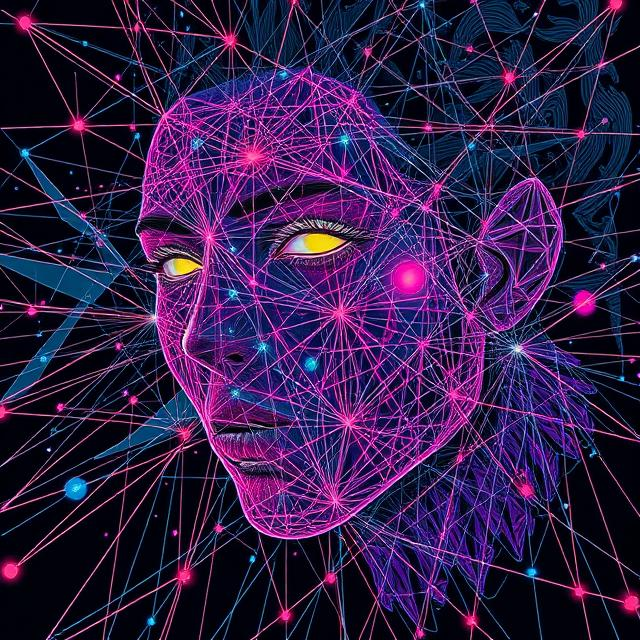
Alperen Keles discusses the ideas of verifiability in software engineering and how it is becoming a limiting factor for utilizing language models (LLMs) to automate programming tasks. LLMs have shown potential in generating code, but the issue lies in verifying its correctness.
The verification process cannot be easily offloaded to LLMs, and even if tests or proofs are generated, their correctness needs to be confirmed by humans.
Alperen Keles argues that for LLMs to succeed in automating programming tasks across all domains, a better focus is needed on improving the tools and interfaces for verifying code. This includes developing new methods of testing software and increasing awareness about non-functional properties such as performance, security, accessibility, and flexibility. The article also highlights the challenge of finding perfect oracles for correctness feedback, but believes that LLMs could potentially outperform humans in this domain if they are capable of producing imperfect but still effective proofs.
Ideas: How OpenAI is building its moat

Ben Dickson's article highlights how OpenAI is transitioning its strategy from relying solely on powerful models to building a moat around application and integration layers. Ben explains that AI can shape applications in two ways: by bringing the application to AI or integrating AI into existing apps. ChatGPT exemplifies the former, evolving from a simple chatbot to an advanced AI tool with various features.
OpenAI's approach of making AI integrate seemlessly into people's dialy lives is illustrated through its macOS app for ChatGPT, which interacts with other applications and provides additional context. However, OpenAI lacks an operating system or distribution channels, putting it at a disadvantage compared to competitors like Apple, Google, and Microsoft.
Crypto News: Robinhood plus Kalshi to launch prediction markets hub

Robinhood is teaming up with Kalsi to launch a prediction markets hub within its app through its subsidiary Robinhood Derivatives, focusing on politics, economics, and sports.
The new feature is made possible by partnering with Kalshi, a firm that facilitates the prediction markets and associated contracts. Initially available across the US through KalshiEX LLC, a CFTC-regulated exchange, the hub aims to expand prediction coverage. "At the most fundamental level, [prediction markets] are the application of capitalism to the pursuit of truth," Tenev said on X.
GitHub Repo: Node Version Audit.
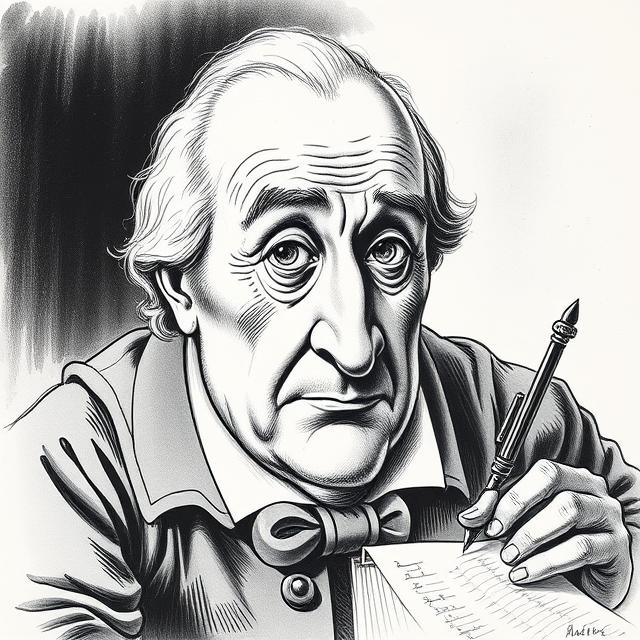
Node Version Audit is a convenience tool to easily check a given Node.js version against a regularly updated list of CVE exploits, new releases, and end of life dates.
It has features such as; List known CVEs for a given version of Node.js, Check either the runtime version of Node.js, or a supplied version, display end-of-life dates for a given version of Node.js, rules automatically updated daily. Information is sourced directly from nodejs.org - you'll never be waiting on someone like me to merge a pull request before getting the latest patch information, Multiple interfaces: CLI (via NPM), Docker, direct code import, easily scriptable for use with CI/CD workflows. All Docker/CLI outputs are in JSON format, Zero dependencies
Ideas: TxAgent: An AI agent for therapeutic reasoning

Precision therapeutics require multimodal adaptive models that generate personalized treatment recommendations. TxAgent, an AI agent that leverages multi-step reasoning and real-time biomedical knowledge retrieval across a toolbox of 211 tools to analyze drug interactions, contraindications, and patient-specific treatment strategies.
TxAgent evaluates how drugs interact at molecular, pharmacokinetic, and clinical levels, identifies contraindications based on patient comorbidities and concurrent medications, and tailors treatment strategies to individual patient characteristics, including age, genetic factors, and disease progression.
TxAgent retrieves and synthesizes evidence from multiple biomedical sources, assesses interactions between drugs and patient conditions, and refines treatment recommendations through iterative reasoning. It selects tools based on task objectives and executes structured function calls to solve therapeutic tasks that require clinical reasoning and cross-source validation. The ToolUniverse consolidates 211 tools from trusted sources, including all US FDA-approved drugs since 1939 and validated clinical insights from Open Targets.
TxAgent outperforms leading LLMs, tool-use models, and reasoning agents across five new benchmarks: DrugPC, BrandPC, GenericPC, TreatmentPC, and DescriptionPC, covering 3,168 drug reasoning tasks and 456 personalized treatment scenarios. It achieves 92.1% accuracy in open-ended drug reasoning tasks, surpassing GPT-4o by up to 25.8% and outperforming DeepSeek-R1 (671B) in structured multi-step reasoning.
TxAgent generalizes across drug name variants and descriptions, maintaining a variance of <0.01 between brand, generic, and description-based drug references, exceeding existing tool-use LLMs by over 55%.
By integrating multi-step inference, real-time knowledge grounding, and tool- assisted decision-making, TxAgent ensures that treatment recommendations align with established clinical guidelines and real-world evidence, reducing the risk of adverse events and improving therapeutic decision-making.
Ideas: Joe Carlsmith - Paths and waystations in AI safety

Joe Carlsmith's article discusses the alignment problem, which refers to the challenge of ensuring that advanced artificial intelligence systems remain aligned with human values and safety goals.
The author suggests distinguishing between two main components: the problem profile (the technical parameters relevant to the alignment problem set by Nature) and civilizational competence (how our civilization responds adequately to a given version of the problem).
Three key security factors are highlighted, including safety progress, risk evaluation, and capability restraint. The discussion also covers different sources of labor and various intermediate milestones that strategies in this respect could focus on. The essay aims to set up further discussions about using future AI labor for AI safety and automating AI alignment.
Comments